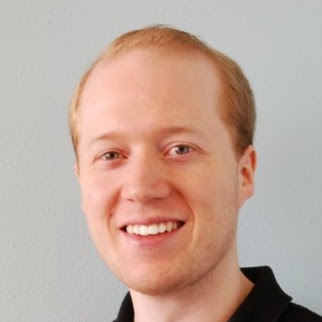
Recently Wang et al. published a paper in the Journal of Experimental Psychology on pre-registered analyses and the use of covariates in un-registered, exploratory, post-hoc analyses, specifically in the context of randomized experiments 1.
I am in 100% agreement with the vast majority of what the authors have to say, and also with the general sentiment of the paper, which I summarize as follows:
1.) Pre-register your analyses and report the results of your pre-registration fully
2.) If you decide to run an unregistered analysis, mark it as exploratory
This is pretty much exactly what I teach my students, what I tell my collaborators, and what I try to do myself in all of my own projects. I think it is wonderful that this is so clearly expressed in this paper, because it’s an important message that deserves to be spread.
Wang et al. then continue to discuss how adding a covariate (preferably one that is strongly related to the outcome) in a data-dependent fashion influences both Type I error and power (hence Type II error). They are only considering a situation in which the treatment was randomized, but in which the inclusion of the covariate was not pre-registered, and is thus exploratory, data-dependent (because it is only done if the unadjusted effect is not significant), and post-hoc. Their argument is simple and straight-forward: the inclusion of such a covariate can greatly decrease Type II errors (because power is increased), and will only increase Type I errors a bit (they are increased because a data-dependent additional analysis is performed). They quantify these errors in a simulation study, and pitch this strategy as one of smart post-hoc exploration of an insignificant result. I think it is also fair to say that they elevate this particular strategy to a special place in all of the exploratory analyses that could have been done. They note e.g., in a supplement that also including the interaction of the covariate and the treatment might not be a good idea, because Type I error inflation seems too high.
Where exactly is my objection? It’s based on a possibly overly strict desire of keeping exploratory and confirmatory research separate from each other. I feel that in Wang et al.’s recommendation they introduce essentially a gray area between the two. To be completely fair, they explicitly note that one should label pre-registered results as confirmatory, and post-hoc as exploratory results, but I think it is also fair to say that they do give a special place for this one particular technique (the estimation of a covariate-adjusted effect using the linear term of a covariate). They consider it a smart way to do a data-dependent post-hoch, exploratory analysis. An applied researcher could easily interpret this as follows: If my original pre-registered analysis does not yield p < .05, then Wang et al. tell me that I now have ONE, and only one, additional shot at p < .05, which is to include a single covariate. If I get p < .05 in that analysis, I still label it as exploratory, but this is somewhat more sanctified than if I had done a whole bunch of other analyses, and it somehow edges more closer to the confirmatory realm.
I would recommend (just as Wang et al.) to label pre-registered results as confirmatory tests of hypotheses. All exploratory results should be labeled and treated as exploratory. That means if you didn’t find the desired effect in your pre-registered results, and only find it once you adjust for a covariate, then this finding should not be used to imply confirmatory evidence for your hypothesis. In addition, and this is contrary to Wang et al. I would not place any limit on the exploratory analyses that one can engage in, as long as they are reported in full, and all labeld as exploratory. Here is why: conducting an exploratory analysis implies just that - being exploratory. Once you are not in confirmation mode anymore, you should be encouraged to be exploratory. Whatever results you find, do not treat them as hypothesis-conforming, but hypothesis-generating, and if possible, follow them up with a replication. As an example, Wang et al. note that the interaction between the covariate and the treatment should not be probed in a data-dependent fashion. What, however, if this interaction is part of the data-generating model (“the ground truth”)? This is exactly what we would like to find in an exploratory fashion - and then, of course, follow-up with a pre-registered replication for any effect that we found.
One thing I should mention is that there might be a misconception among researchers that once you have a pre-registration in place, your hands are tied not to do any other analysis (Wang et al. describe such a person who never conducts any additional analyses in their paper). I am not sure how widespread this belief is, but there is really nothing that prevents a researcher from doing additional, exploratory, post-hoc, data-dependent, snooping analyses (as long as they are labeled exploratory and are reported in full!). The pre-registration “only” provides a demarcation line between hypothesis-conforming and hypothesis-generating research, prevents HARKing (Hypothesizing after results are known), and keeps researchers honest. So if this is a widespread belief, then the Wang et al. paper is again doing a great job of dispelling it.
P.S. Uli Schimmack actually beat me to this conclusion, and posted it on the Psych Methods Facebook page…
While I don’t know the authors of this paper, for full disclosure, I should mention that the senior author of this paper, Alison Ledgerwood, was the editor on a paper of mine for Perspectives on Psychological Science. She was a great editor. I felt it’s important to express this here, because if anything, I am positively biased against her, and I am also not directing my criticism directly at her as a person.↩